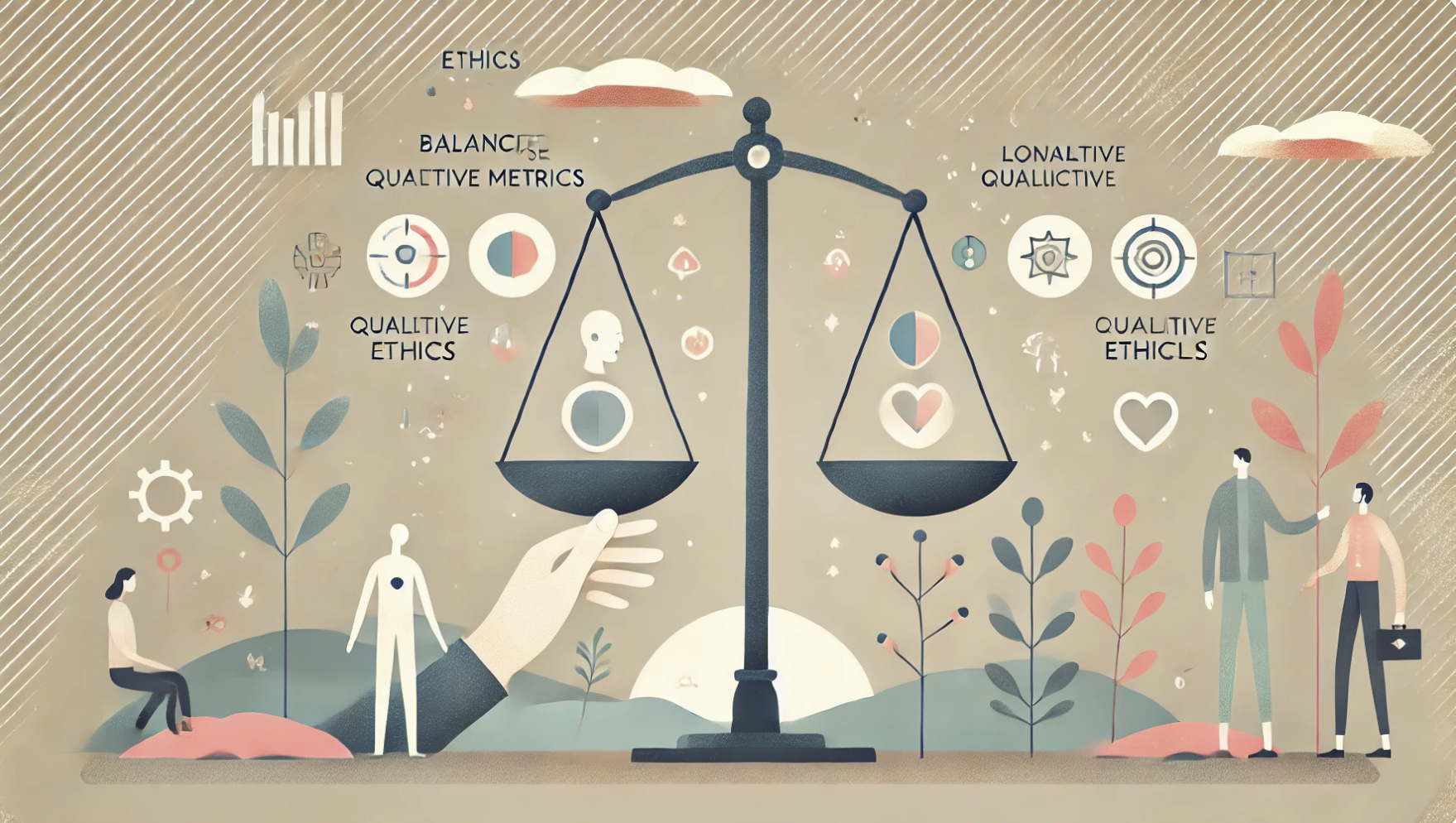
Goodbye Goodhart: Escaping metrics and policy gaming in Responsible AI
As Responsible AI (RAI) program implementations mature over time, they often introduce metrics to track how well the organization is doing in terms of its RAI posture. Additionally, to motivate adoption by staff, there are often incentives that are either introduced or altered within existing functions to ensure that there is alignment towards the program’s goals and progress is made steadily towards achieving those goals. Yet, there is a common pitfall that any such approach is susceptible to: Goodhart’s Law, i.e., “When a measure becomes a target, it ceases to be a good measure.”
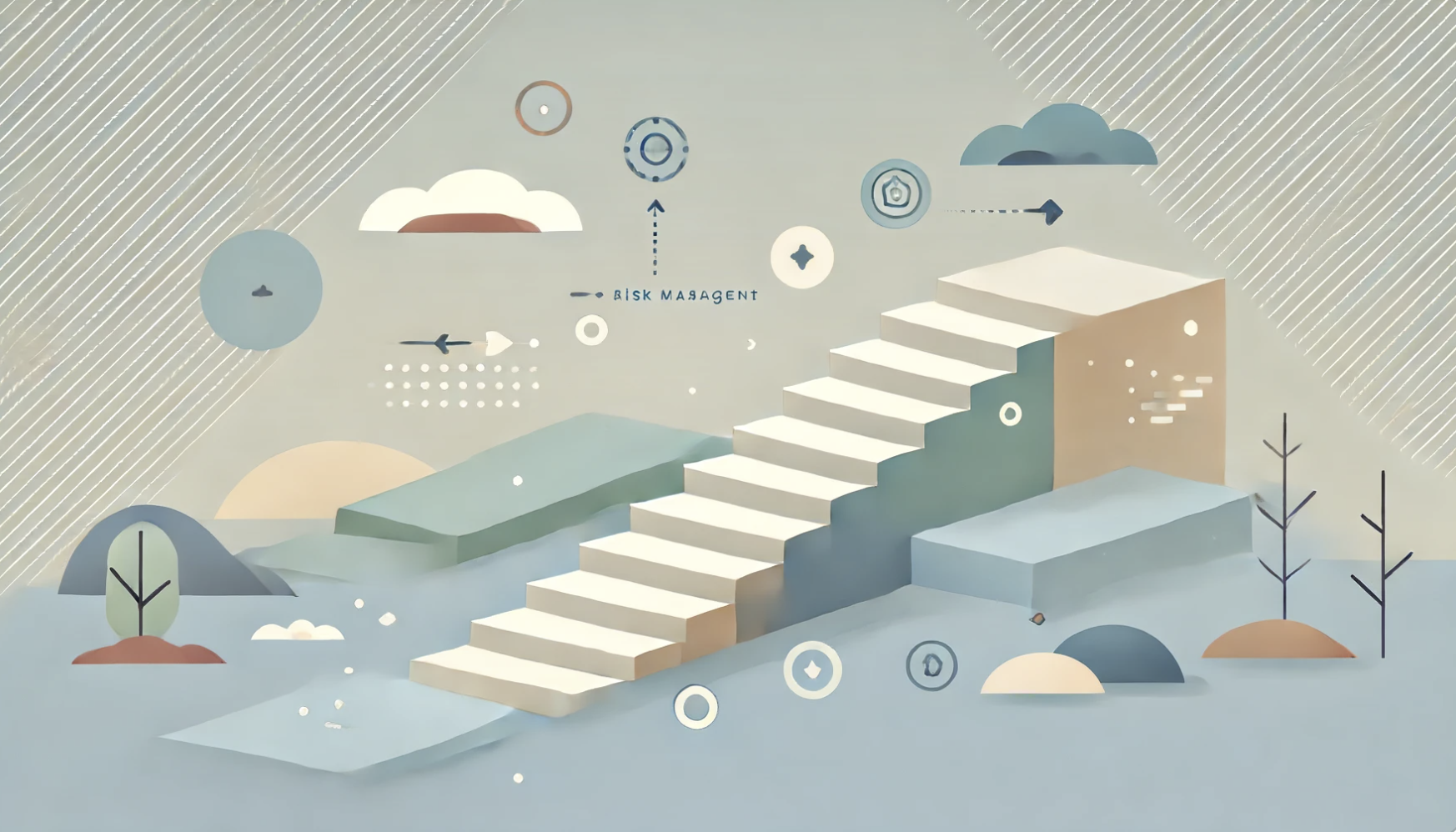
Making large changes in small, safe steps for Responsible AI program implementation
The "large changes in small safe steps" approach leads to more successful program implementation by effectively mitigating risks, enhancing stakeholder engagement and trust, and ensuring sustainable and scalable adoption of new practices. This strategic method balances innovation with caution, fostering a resilient and adaptive framework for Responsible AI programs.
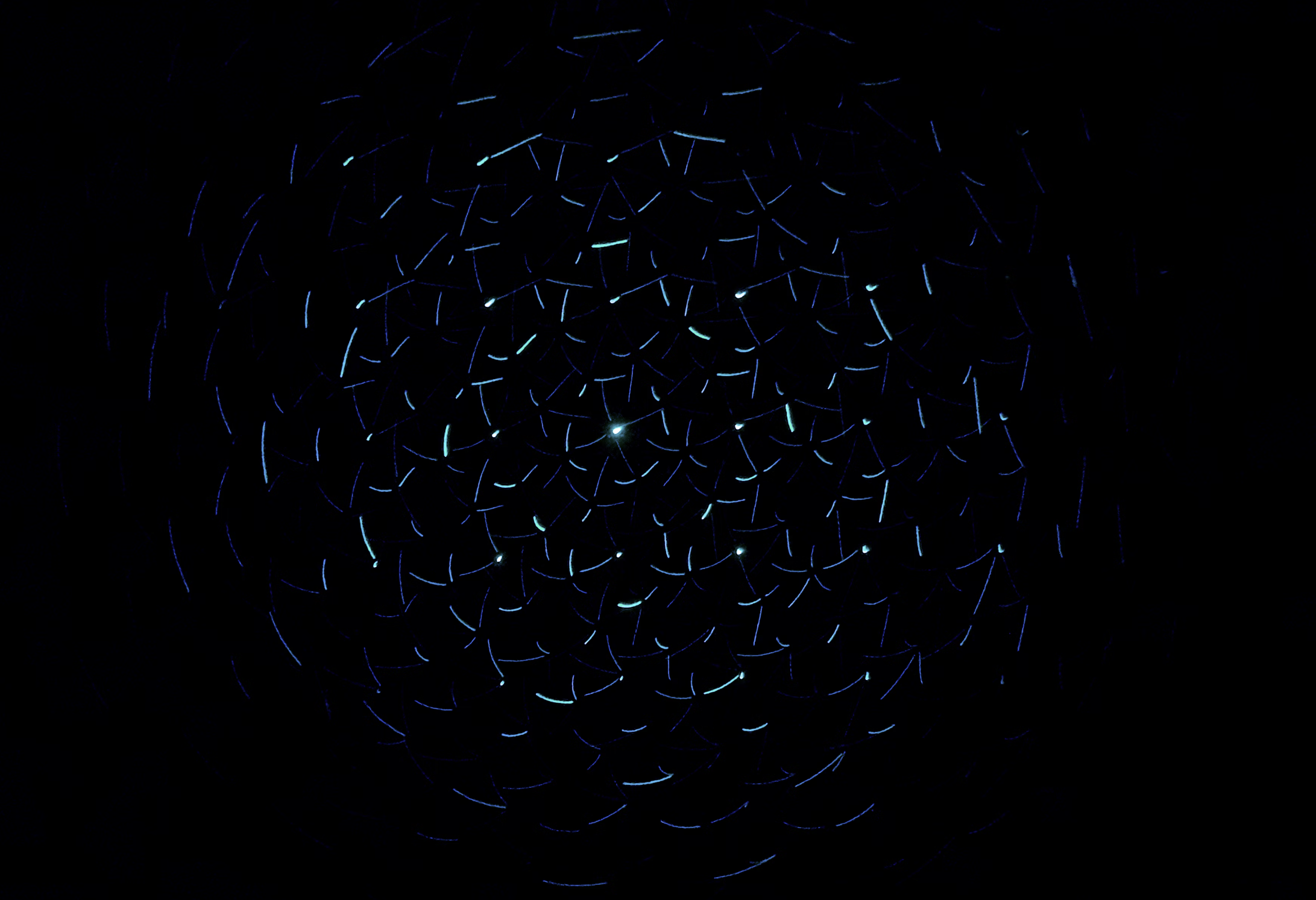
Think further into the future: An approach to better RAI programs
As artificial intelligence (AI) continues to permeate various aspects of society, the urgency to implement robust Responsible AI (RAI) programs has never been greater. Traditional approaches often focus on immediate risks and foreseeable consequences, but the rapidly evolving nature of AI demands a more forward-thinking strategy. To truly safeguard against ethical pitfalls and unintended consequences, organizations must adopt a mindset that looks further into the future, predicting the far-reaching impacts of current trends.
Let’s look at a framework I use in my advisory work grounded in (1) proactive risk assessment, (2) scenario planning, and (3) ethical foresight. By extrapolating further than conventional methods and anticipating potential scenarios that might initially seem improbable, organizations can build AI systems that are responsible today and resilient to future challenges. The goal is to create AI governance structures that are adaptable, inclusive, and prepared for a wide range of outcomes.
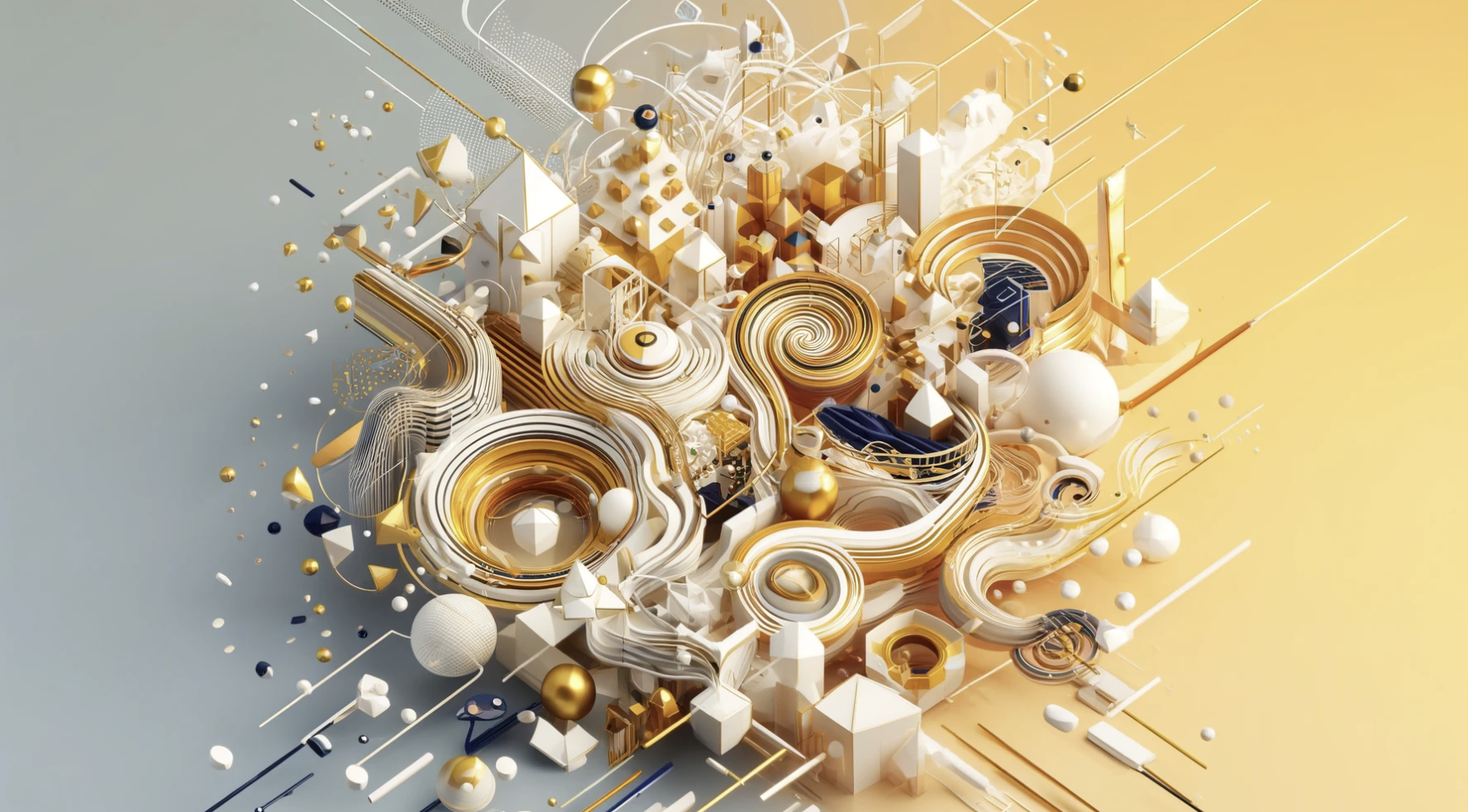
Keep It Simple, Keep It Right: A Maxim for Responsible AI
The path to Responsible AI (RAI) is often mired in complexity, leading to challenges in transparency, accountability, and trust. Amidst this complexity, a timeless principle offers a guiding light: simplicity.
The simplest way of doing something is often the right way. This maxim not only holds true in life but is also a powerful strategy for designing and implementing RAI programs. By embracing simplicity, we can create AI systems that are easier to understand, maintain, and govern—ultimately ensuring they align with ethical standards and societal values.
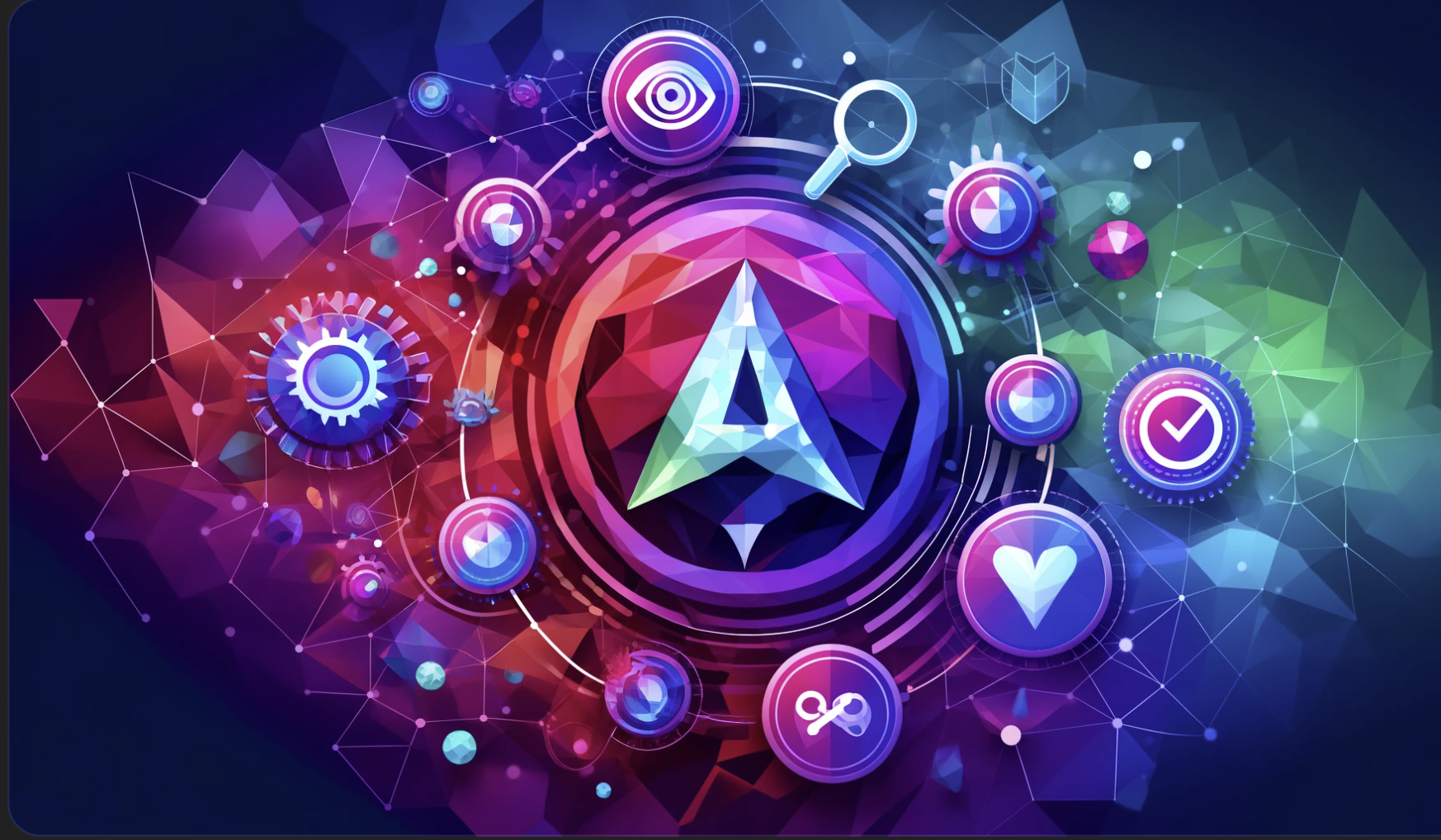
A guide on process fundamentals for Responsible AI implementation
In the rapidly evolving AI landscape, operationalizing Responsible AI (RAI) is not just a technical challenge but a fundamental organizational imperative. As AI systems increasingly influence societal outcomes, ensuring they are developed and deployed ethically requires a nuanced approach that integrates robust management practices with a deep understanding of both technological and human factors.
Let’s explore a structured process for implementing RAI changes, emphasizing the importance of aligning authority and responsibility, fostering collective ownership, and designing safe and adaptable processes. By adopting these principles, organizations can navigate the complexities of RAI, ensuring their AI initiatives are innovative and aligned with broader ethical and societal values.
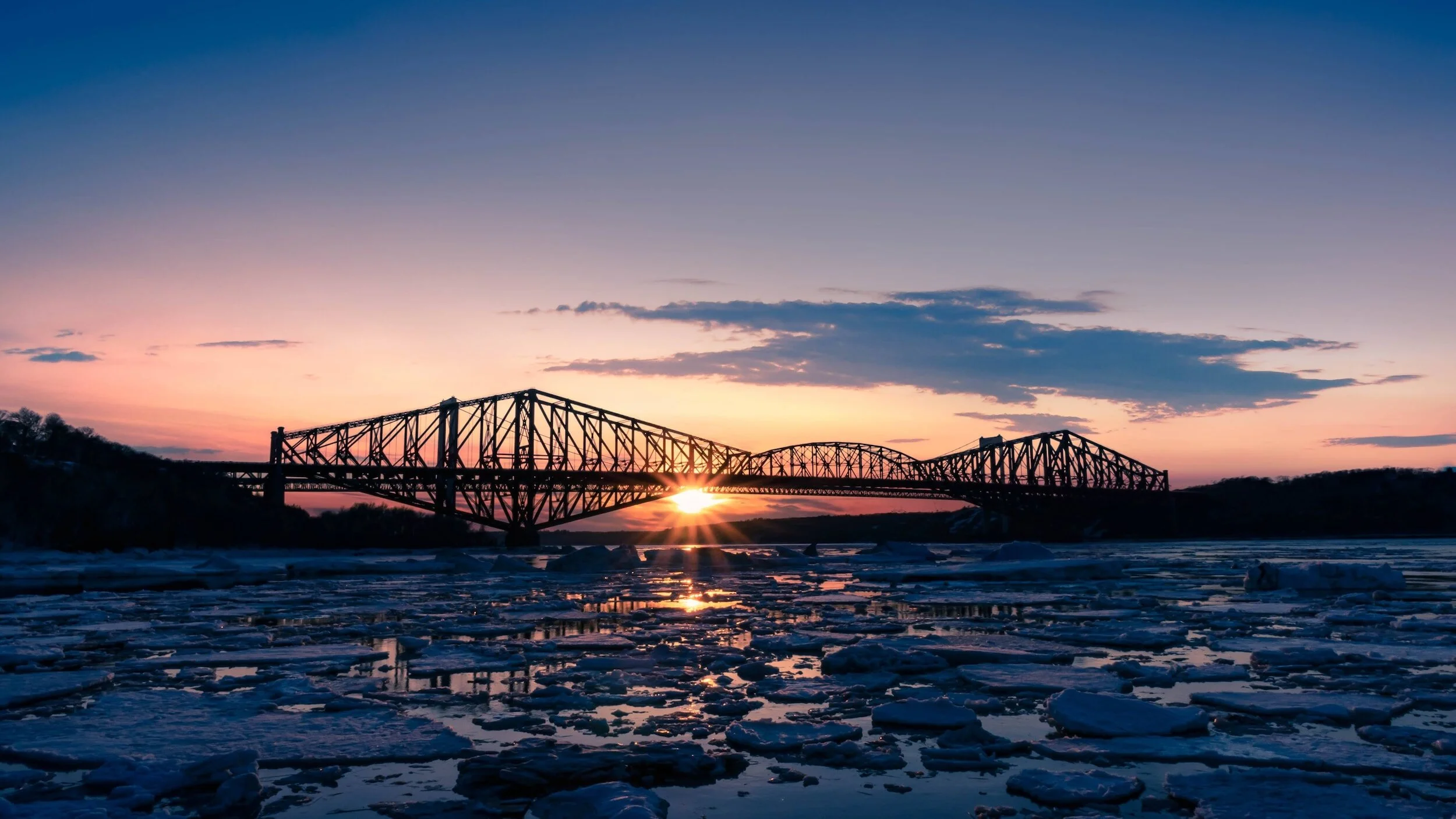
The Future of AI in Quebec: Bridging Gaps to Drive Innovation, Growth and Social Good
Artificial intelligence (AI) is transforming societies and economies around the world at a rapid pace. However, Quebec risks falling behind in leveraging the opportunities of AI due to several gaps in its ecosystem. In this comprehensive blog post, I analyze the current limitations around AI development, adoption, and governance in Quebec across the public, private, and academic sectors. Based on this diagnosis, I then provide targeted, actionable recommendations on how Quebec can build understanding, expertise, collaboration, and oversight to unlock the full potential of AI as a force for economic and social good. Read on for insights into the seven key areas requiring intervention and over 40 proposed solutions to propel Quebec into a leadership position in the global AI landscape.
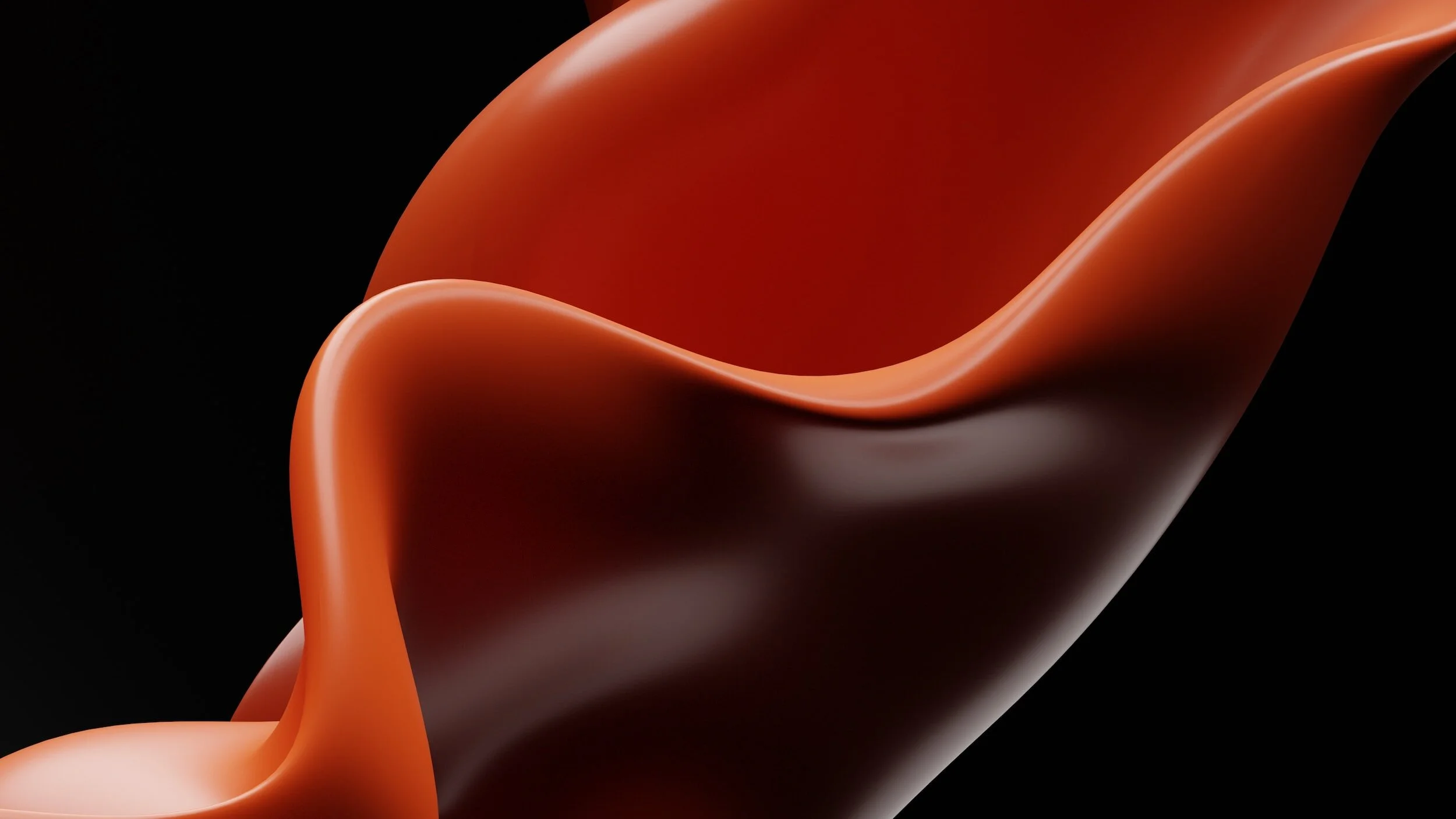
Bridging the intention-action gap in the Universal Guidelines on AI
We are now firmly in a world where organizations are beginning to see returns from their investments in AI adoption within their organizations. At the same time, they are also experiencing growing pains, such as the emergence of shadow AI, that raises cybersecurity concerns. While useful as a North Star, guidelines need accompanying details that help implement them in practice. Right now, we have an unmitigated intention-action gap that needs to be addressed - it can help strengthen the UGAI and enhance its impact as organizations adopt this as their de facto set of guidelines.
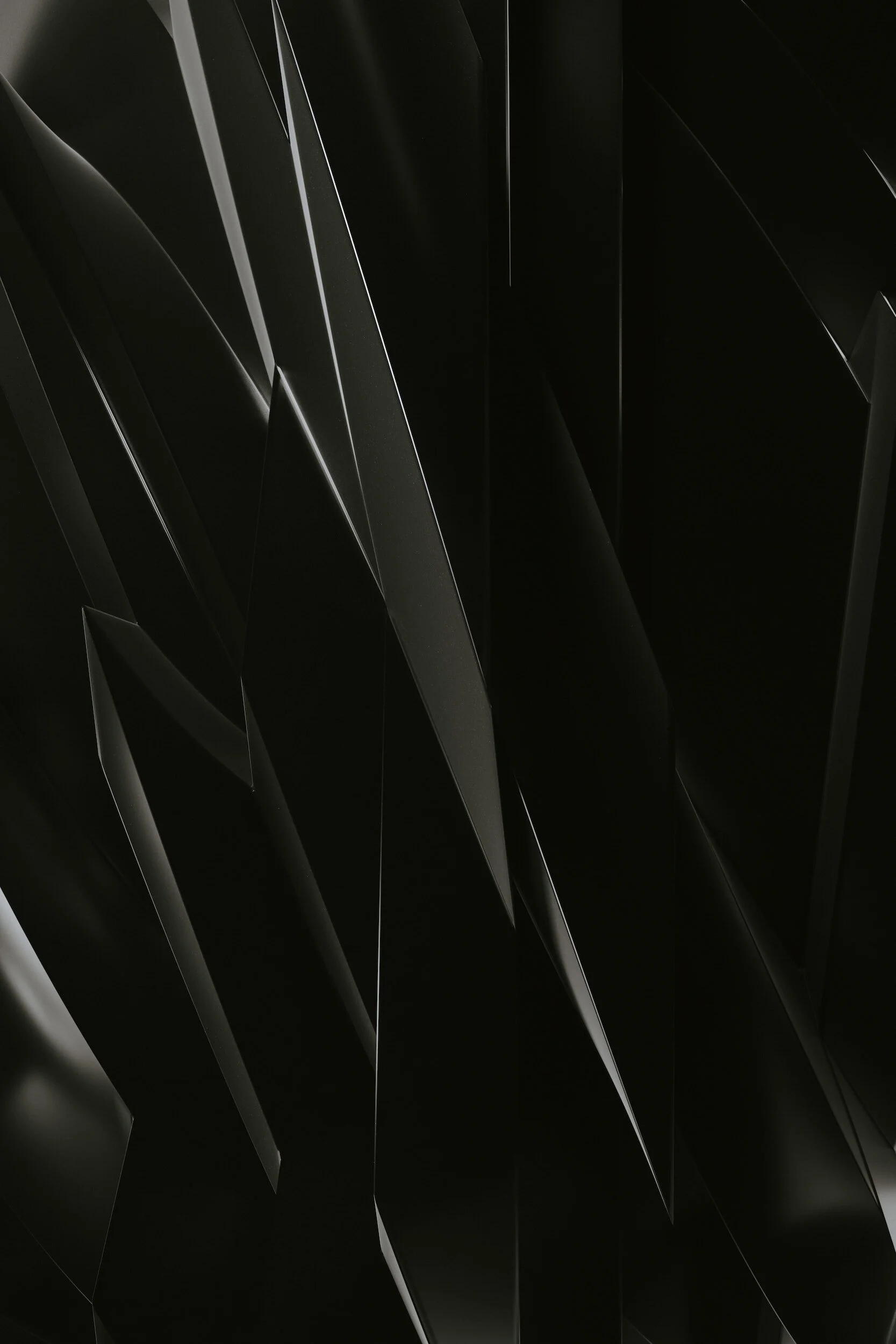
A Research Roadmap for an Augmented World
At a granular level - what skills may be valued in a human-machine economy? We don't have the answers but hope to define the problem today, bring in examples, and outline a collaborative research agenda so the community's collective intelligence (CI) can progress. That way, leaders will have a glimpse ahead with better questions, so the transition is less bumpy.
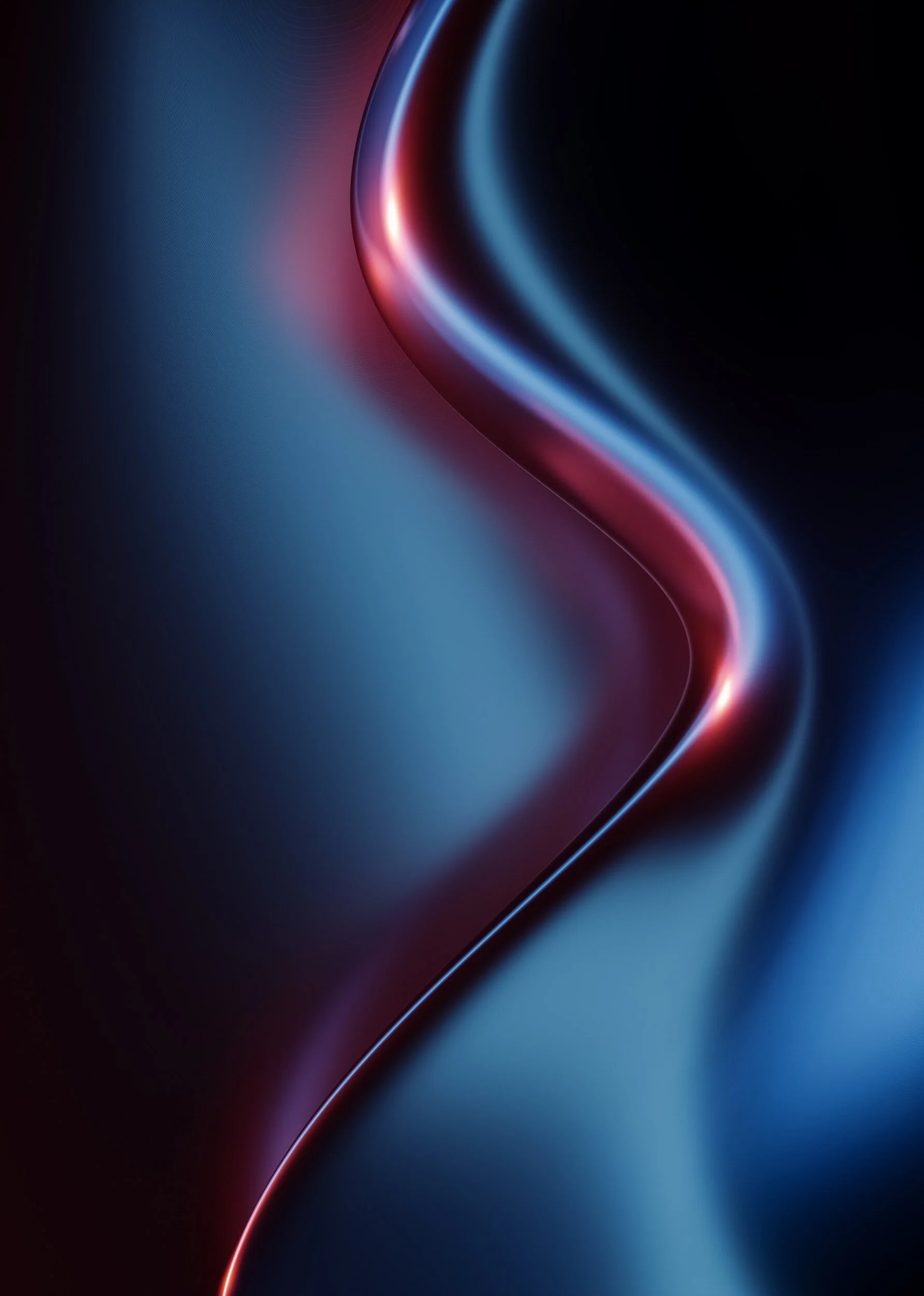
Good futurism, bad futurism: A global tour of augmented collective intelligence
This fall, Abhishek Gupta and I are rolling our insights into a series of experiments. (If you’d like to be a human volunteer, send me a note!) It is our hope to understand not just the principles underlying ACI but to catch it in action in a hybrid human-AI team exercise. We want our legacy to be a set of stepping stones towards a greater understanding of human-AI teaming, its risks and benefits, and how responsible organizations can implement large language models (LLMs) in their daily work.
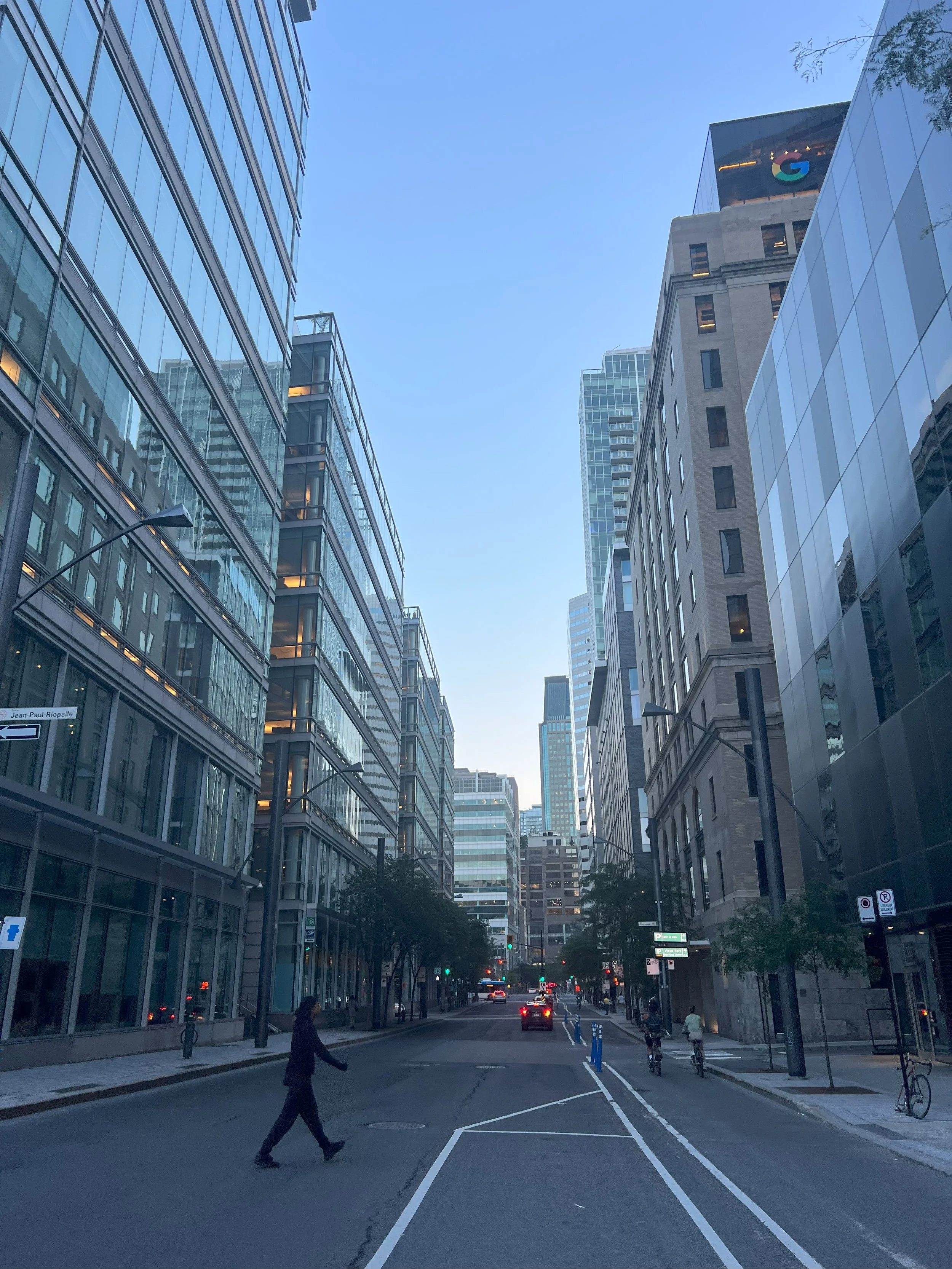
Seeing the invisible: AIES 2023 - Day 3
One way to think of AI is as “invisible work.” By design, it performs tasks that humans would otherwise complete with invisible effort. It can become easy to stop asking how it was made or with whose data. Today at AIES, we’re talking about how it takes a village to raise an AI system and learn what that village needs.
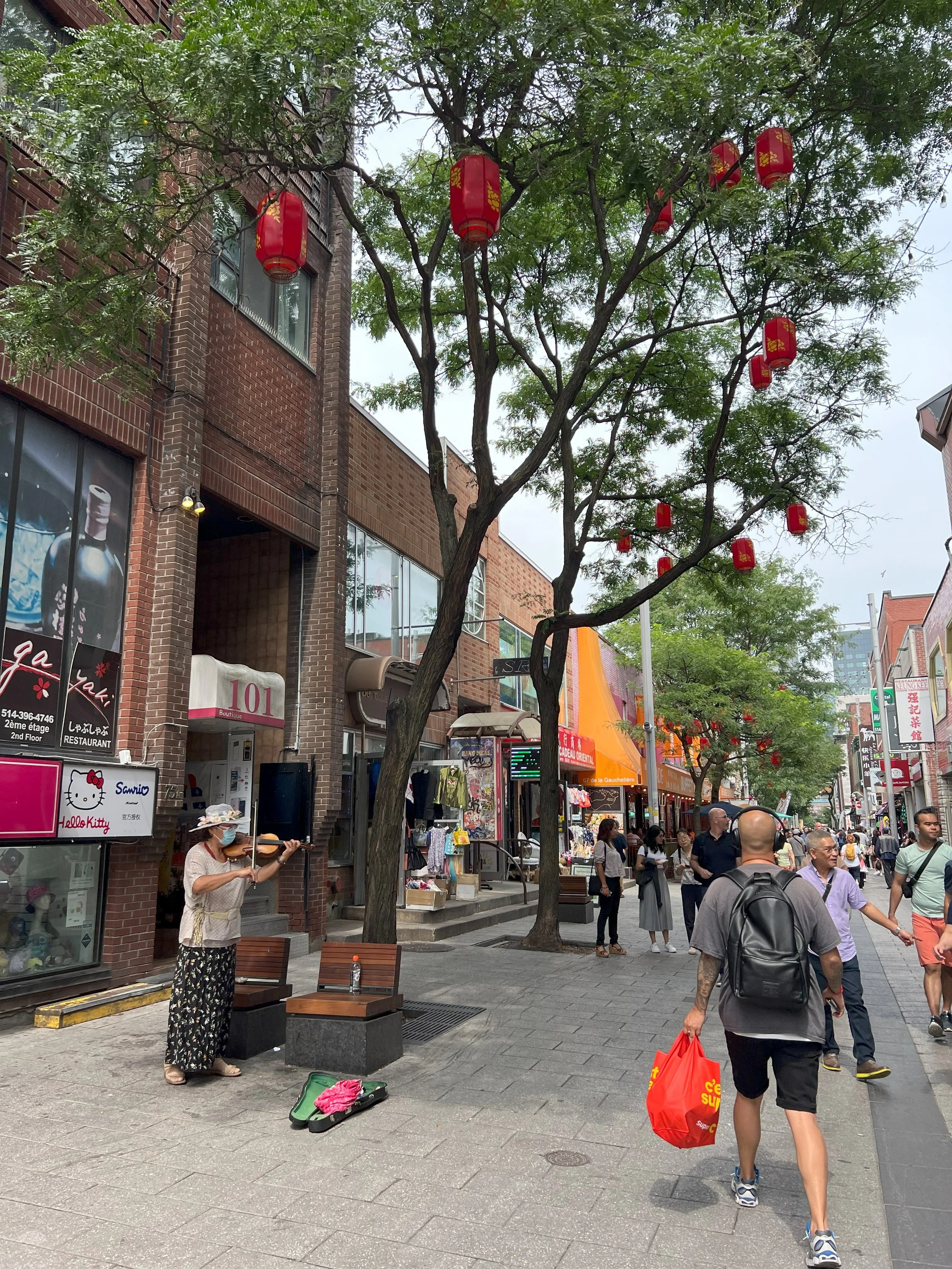
Democratizing AI: AIES 2023 - Day 2
We think of bias in machine learning, like we do about people. We’re biased or unbiased; we’re corrupt, or we’re pure. The beauty and irony of machine learning lie in how difficult it is to make an orderly representation of our will when our will is anything but orderly. Bias mitigation is not a one-stop shop. It’s hard, and sometimes our efforts backfire. Today, we’re looking at the old problems behind our newest technology.
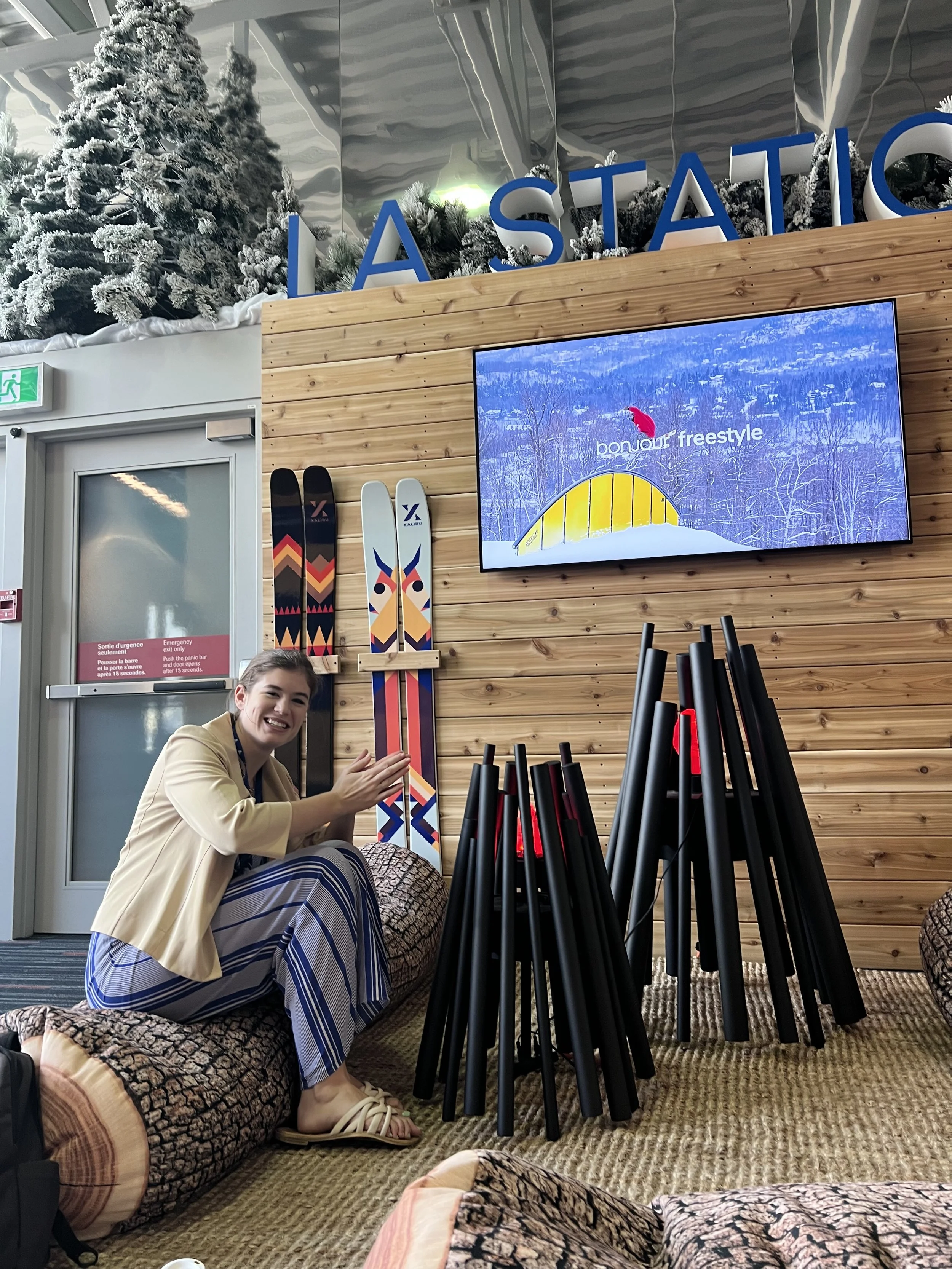
Deciding who decides: AIES 2023 - Day 1
Shifting the AI governance paradigm from an oligopoly into something more democratic is how we can all reap the benefits of collective intelligence. We’re here in Montreal this week to learn from the researchers chipping away at each piece of this problem.
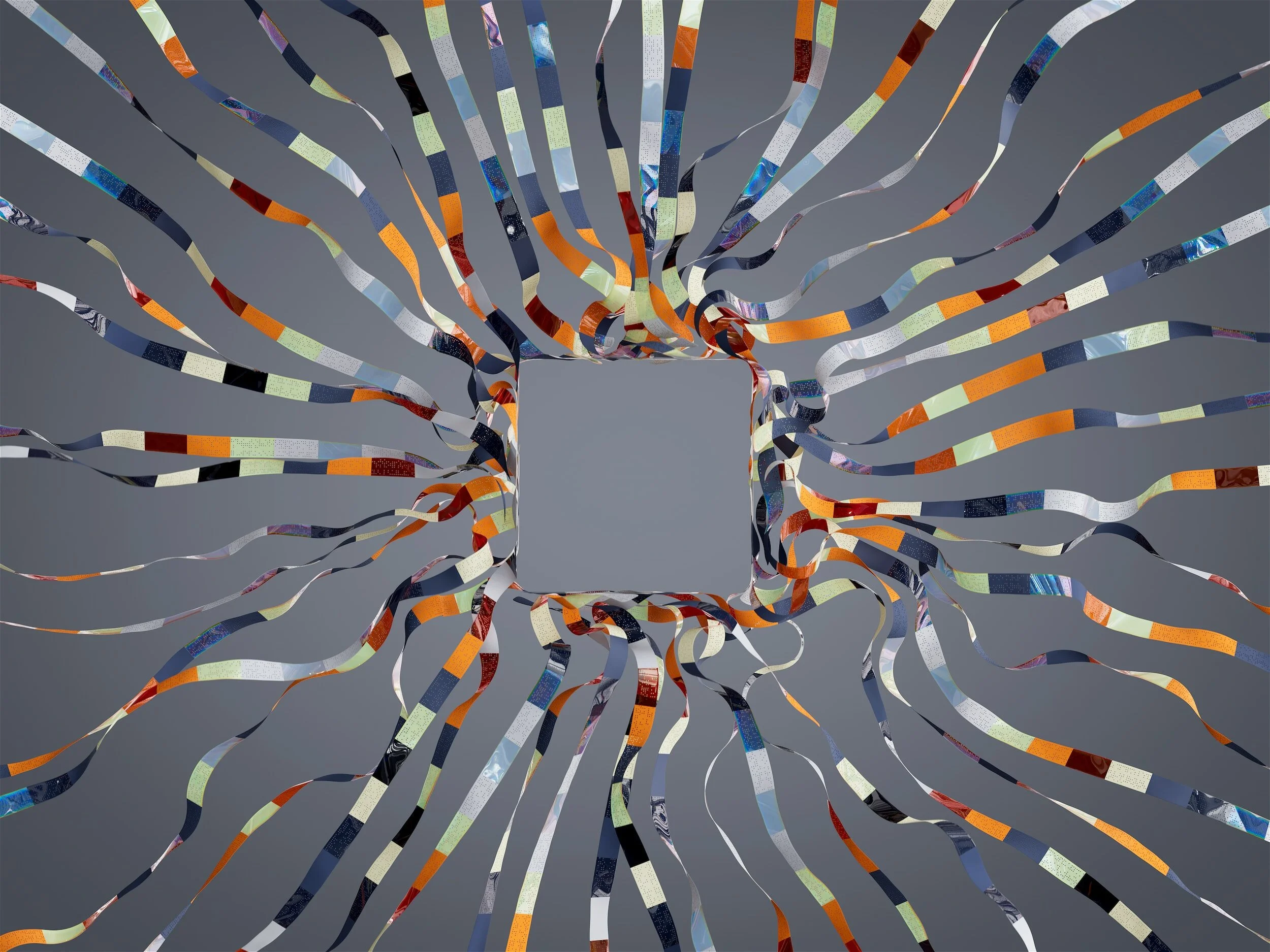
Poor facsimile: The problem in chatbot conversations with historical figures
It is important to recognize that AI systems often provide a poor representation and imitation of a person's true identity. As a reference, it can be compared to a blurry JPEG image, lacking depth and accuracy. AI systems are also limited by the information that has been published and captured in their training datasets. The responses they provide can only be as accurate as the data they have been trained on. It is crucial to have extensive and detailed data in order to capture the relevant tone and authentic views of the person being represented.
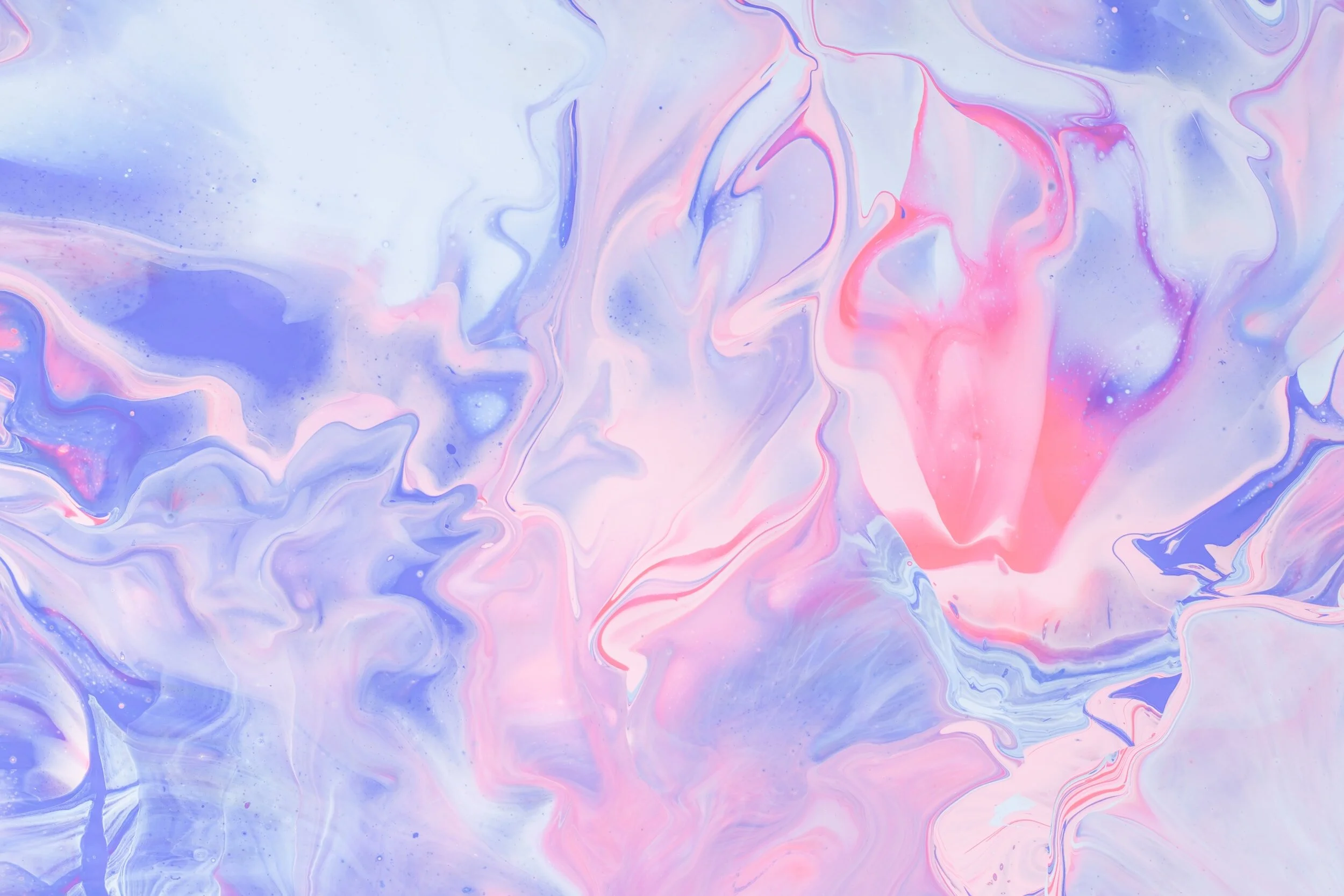
Hallucinating and moving fast
"Move fast and break things" is broken. But we've all said that many times before. Instead, I believe we need to adopt the "Move fast and fix things" approach. Given the rapid pace of innovation and its distributed nature across many diverse actors in the ecosystem building new capabilities, realistically, it is infeasible to hope to course-correct at the same pace. Because course correction is a much harder and slow-yielding activity, this ends up amplifying the magnitude of the impact of negative consequences.
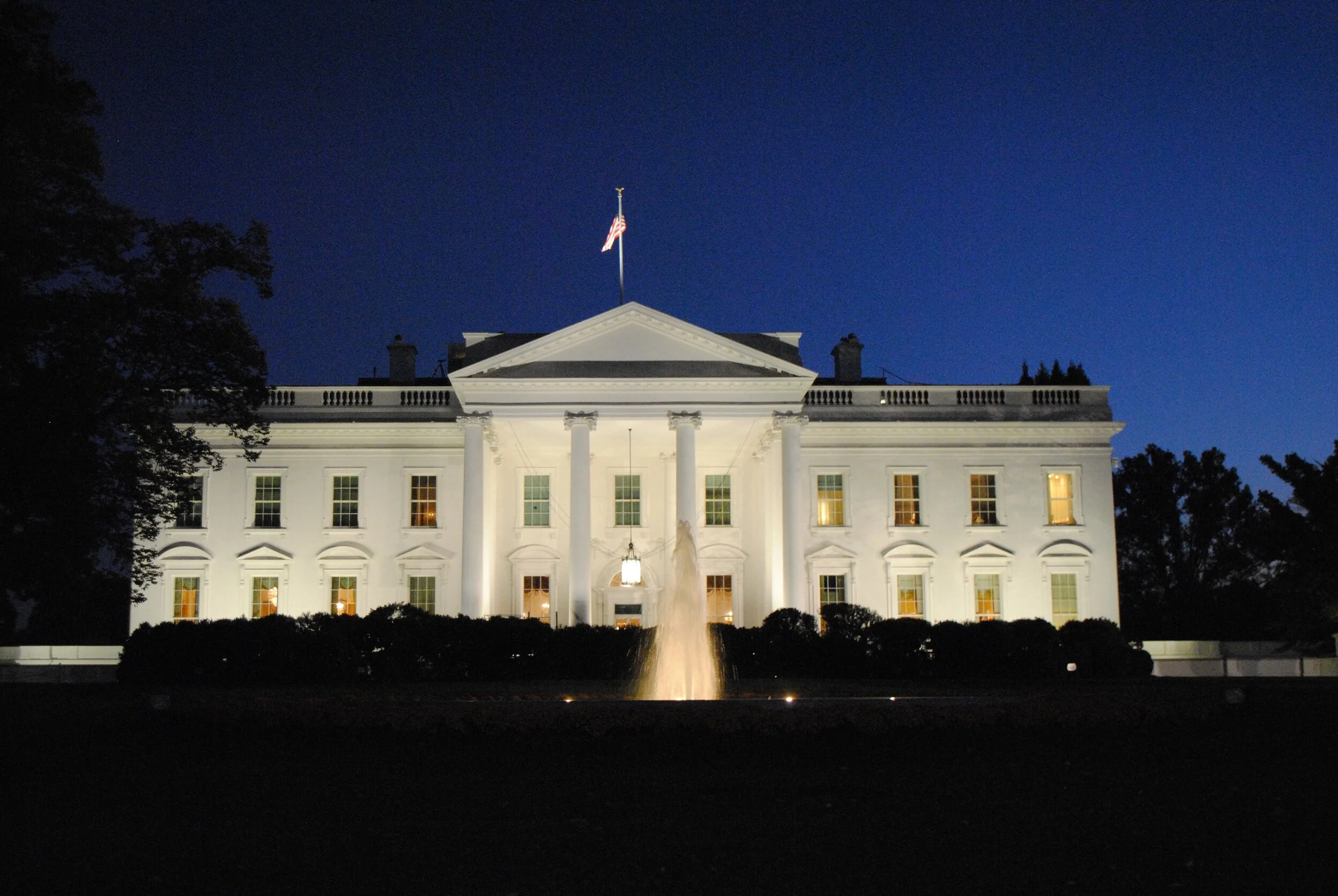
Moving the needle on the voluntary AI commitments to the White House
The recent voluntary commitments secured by the White House from the core developers of advanced AI systems (OpenAI, Microsoft, Anthropic, Inflection, Amazon, Google, and Meta) presents an important first step in building and using safe, secure, and trustworthy AI. While it is easy to shrug aside voluntary commitments as "ethics washing," we believe that they are a welcome change.
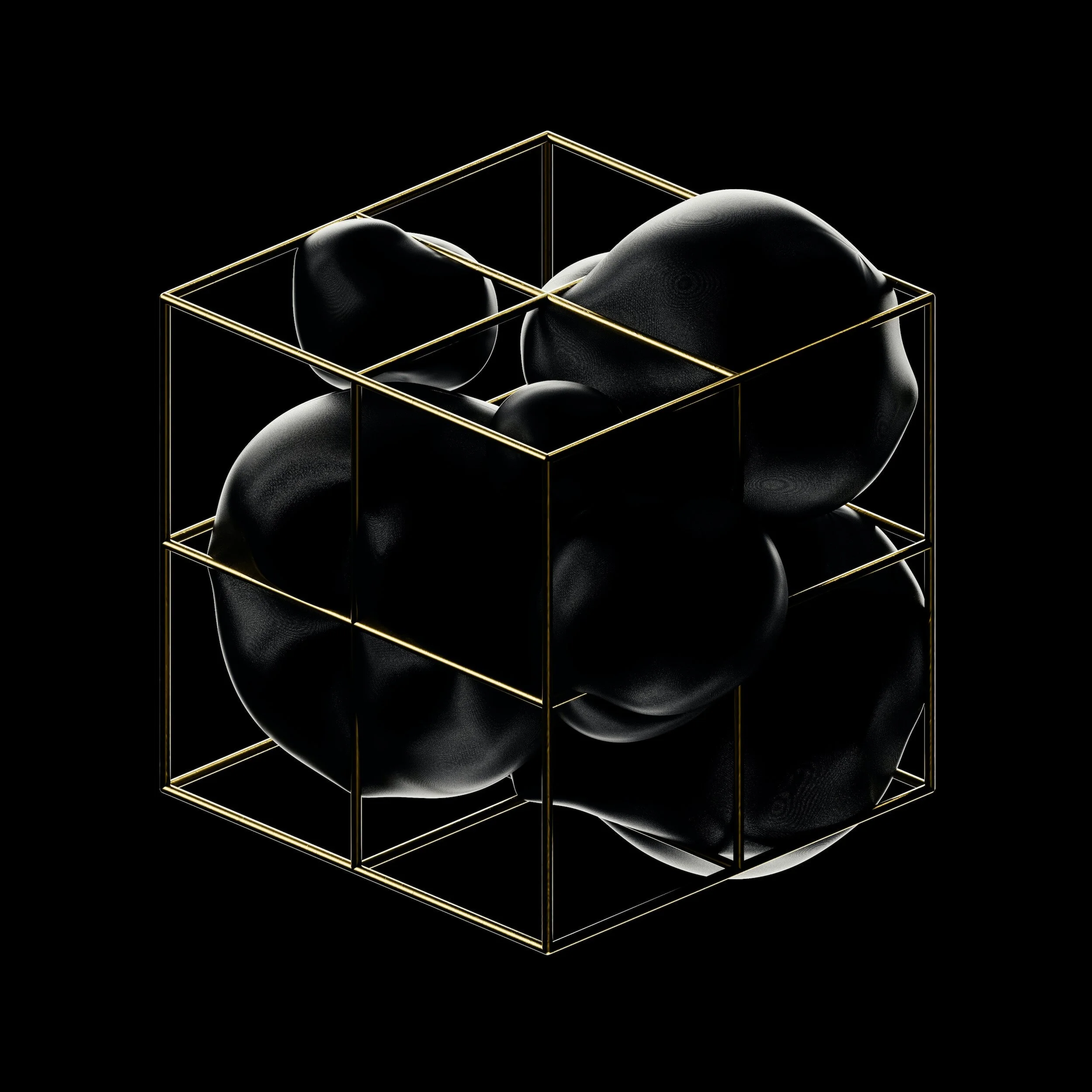
War Room: Artificial Teammates, Experiment 6
Effective communication can make or break a crisis response. Can Anthropic’s new model, Claude 2, facilitate better decision-making in crunch time?
Controlling creations - ALIFE 2023 - Day 5
The advent of artificial life will be the most significant historical event since the emergence of human beings…We must take steps now to shape the emergence of artificial organisms; they have the potential to be either the ugliest terrestrial disaster or the most beautiful creation of humanity.
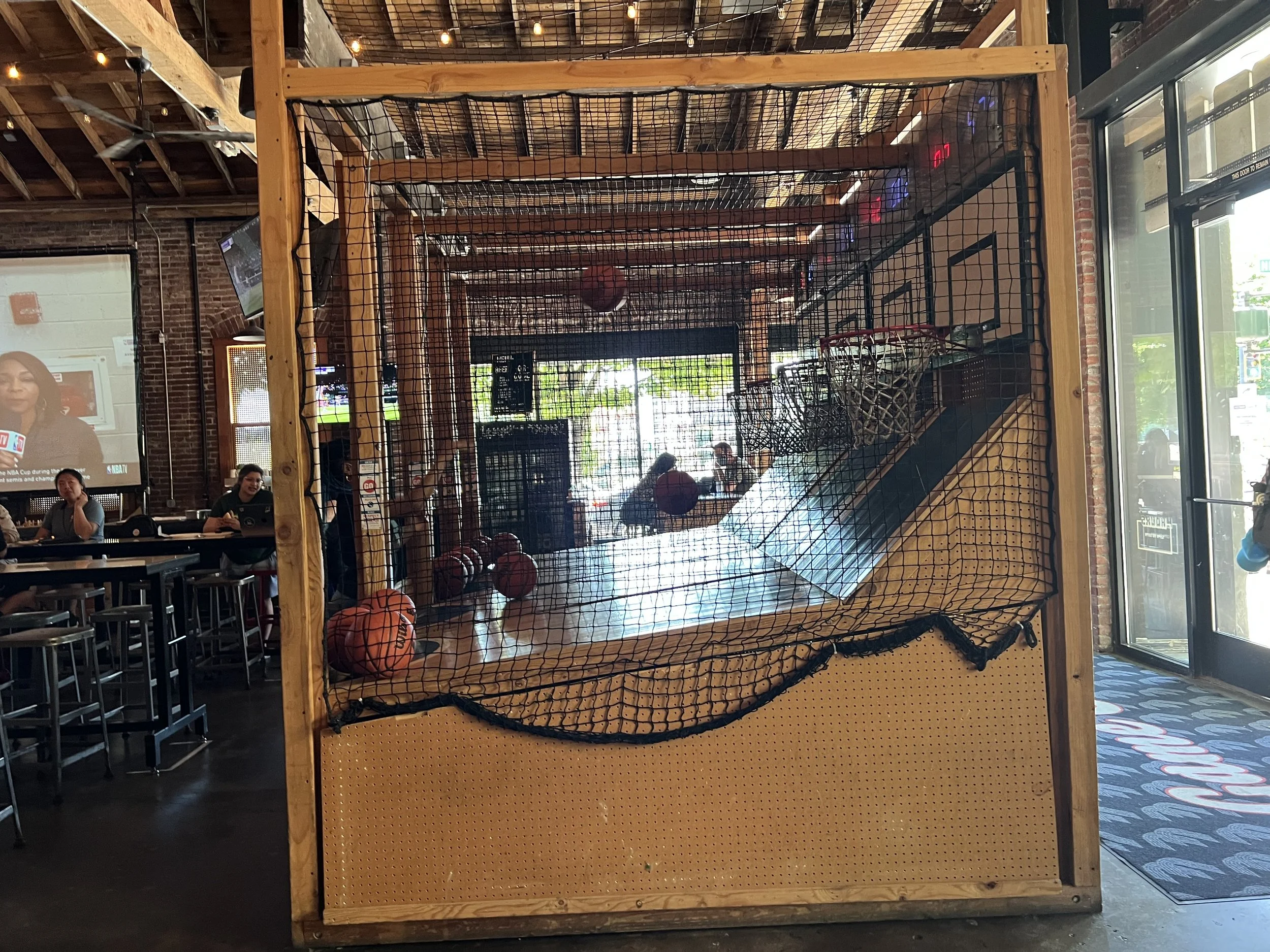
Enabling collective intelligence: FOSSY Day 4
Collective intelligence (CI) runs on contribution, but setting up a system to elicit collective intelligence isn’t easy. Many open-source projects are created and maintained by an individual founder, who sometimes claims the title of “Benevolent Dictator for Life.” It’s halfway a joke, pointing out the inherent tension between a participatory project and the unilateral actions it takes to set one up. Nothing is free – no margin, no mission. But figuring out how to distribute power quickly and effectively is essential to generate CI.
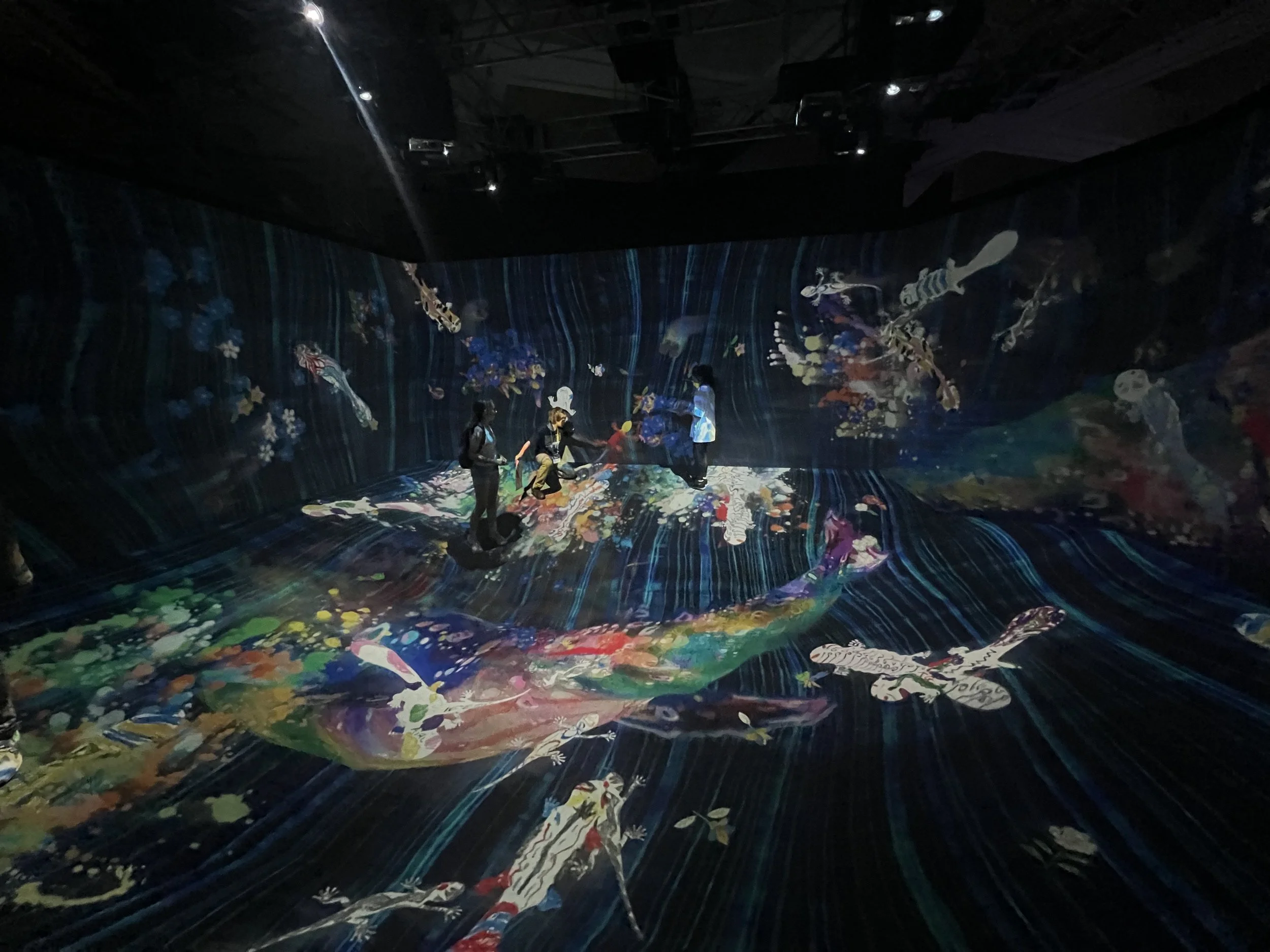
Entropy, measurement, and diversity: ALIFE 2023 - Day 4
In AI, something that excites and worries many researchers is “emergent capabilities” – a phenomenon observed in all complex systems in which complex network interactions lead to entirely new traits and abilities. We build and rely on complex systems in the first place to handle change, but if that system becomes too unpredictable, it stops being useful and might even be dangerous. This is the core problem in science: can we design predictable systems with unpredictable properties? Can we find simple rules and theories to explain complex phenomena without losing their most important parts?
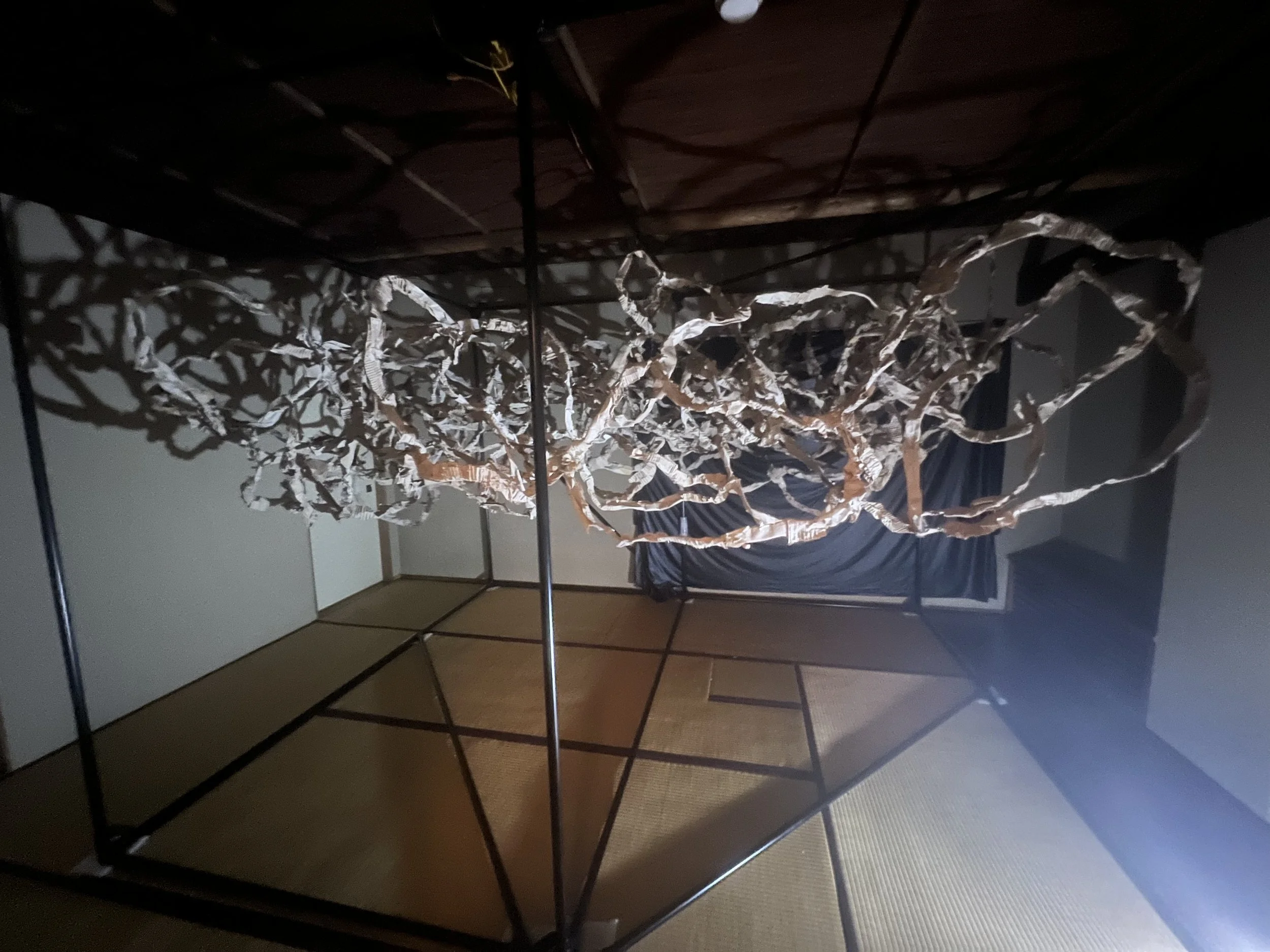
Embodiment and emergence: ALIFE 2023 - Day 3
It might surprise people that artificial life ("Alife") is not a new field – it has deep roots in evolutionary biology, information science, computer engineering, psychology, and art. Alife blends the fanciful with the practical – looming sculptures of wiggling network interactions alongside urgent warnings about how *not* to train humans how to use AIs. To solve the world's toughest problems, it's probably good to start by understanding what makes us 1) intelligent or 2) alive.